Moving forward with Multiple Sclerosis diagnosis
TheraPanacea is proud to share a recent peer-reviewed article published in Neuroimage in partnership with BiogenDigitalHealth describing a new machine learning model that classifies acute vs chronic Muliple Sclerosis (MS) lesions using single time point non-contrast conventional T1- and T2-weighted MRI scans.
Multiple sclerosis is a chronic immune-mediated neuro-degenerative disease affecting the central nervous system and mainly characterized by the accumulation of demyelinated lesions or plaques detectable on magnetic resonance imaging (MRI). Currently, most conventional T2-weighted brain MRI techniques allow for the detection of these pathological hallmarks as white matter hyperintensities (WMH). However, WMHs do not discriminate between MS lesions that may be undergoing active demyelination (known as acute) from lesions that have been present for some time (known as chronic). Today, acute lesion detection on a single time point and in the absence of a prior reference scan relies on the segmentation of hyperintense foci on post-gadolinium T1-weighted MRI, which may underestimate recent acute lesion activity.
Availability of automated methods that are able to differentiate between acute and chronic lesions using conventional MRI may be relevant for the clinical management of patients with MS in guiding treatment decisions, such as switching anti-inflammatory disease-modifying therapies, and may support short- and long-term disease prognostication.
The aim of the research presented in this article was to improve the sensitivity of acute MS lesion detection in the single time point setting by developing a novel machine learning approach for the automatic detection of acute MS lesions using conventional non-contrast T1- and T2- weighted brain MRI. The methods rely on the extraction of textural radiomic features from MRI data, which provide a large number of quantitative features describing the spatial pattern of intensity values within regions of interest on MRI scans. It is hypothesized that textural features are sensitive to microscopic pathological processes in MS and texture analysis of MRI scans has shown promise in lesion classification and prediction and adds interpretability to algorithm output.
The algorithm demonstrated good generalization properties across different MS disease stages (relapsing-remitting MS [RRMS] in ADVANCE/DECIDE clinical trials and secondary progressive MS [SPMS] in ASCEND trial). The model achieved 75.8% balanced accuracy on a validation set of RRMS subjects, which was maintained on independent test datasets comprising data from both SPMS (74.6% accuracy) and RRMS (74.3% accuracy) populations.
Future work will focus on evolving the voxel-level MS lesion classifier to a brain-level segmentation, which would augment current methods, and to assess the relationship between acute lesion burden (defined using the classifier) with subsequent clinical disease outcomes. Implications of the development of this classifier include the potential elimination of the need for gadolinium injection and improved prognostication.
These results illustrate how an automatic algorithm using standard MRI sequences as input can lead to voxel-level maps of predicted acute MS lesion activity that may support diagnosis and prognostication of MS.
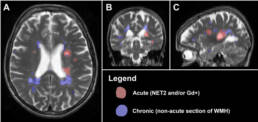
Figure. Axial (A), coronal (B), and sagittal (C) views of a T2-weighted brain MRI scan randomly selected from the ADVANCE cohort, showing the acute (red) and chronic (blue) ground truth segmentation maps. We observe isolated chronic and acute foci, as well as contiguous acute and chronic lesions.
Want to learn more about this publication?
Related Posts
25 June 2024
Unlocking the Potential of AI Auto-Contouring in Radiotherapy at HELSE Bergen [WEBINAR]
Clinically Relevant AI in Radiation…
24 October 2023
Innovations in Radiation Oncology: Harnessing AI for better patients outcomes [WEBINAR]
Clinically Relevant AI in Radiation…